April 20, 2023
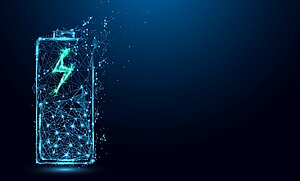
The Fraunhofer Heinrich-Hertz-Institut (HHI) is coordinating the new project KI2L (real-time detection of health-state-relevant processes in lithium-ion batteries through AI-supported characterization for the resource-efficient development of after-use potential). As part of the project, researchers are developing an AI-supported characterization of lithium-ion batteries. Through new characterization possibilities, safety-critical wear processes inside the battery cell are detected reliably so that damage to the entire compound can be avoided in further operation. In accordance, more lithium-ion batteries can be used successfully as second-life components. KI2L started in January 2023 and will run for three years until January 2026. The project is funded by the Federal Ministry of Education and Research (BMBF) with two million euros as part of the BMBF initiative "Battery 2020 Transfer." Fraunhofer HHI will receive 450,000 euros of the overall amount.
Meaningfully reducing the CO2 footprint of lithium-ion batteries demands maximum use as second-life batteries. To ensure that as many discarded battery cells as possible can be utilized in so-called 're-use scenarios,' companies need rapid characterization methods and intelligent decision-making support for concrete re-use potentials. Furthermore, the industry requires automated disassembly process steps so that they can effectively replace defective storage components.
Currently, experts record the condition of lithium-ion batteries by testing the discharge capacity under specific conditions such as battery temperature and discharge current. However, as the batteries age, this procedure becomes error-prone and time-consuming.
The KI2L project team has set itself the goal of improving the condition recording process with an AI-supported characterization. In the first step, the researchers will analyze both as-new and real-scenario tested pre-aged lithium-ion battery cells, equipping them with an optical temperature and strain field measurement system at the module level. Subsequently, targeted characterization methods are employed to identify variances and recurring patterns in the measurement signals of the differently aged battery storage units to precisely record changes in real-time using state sensors. The data obtained is stored on a blockchain in an aging process database and used to train neural networks. An AI trained in this way can identify irreversibly aged cells in real storage operation by means of rapid analysis and remove them by laser processing without causing any overall damage to the battery, thus extending the life cycle of the batteries.
Jointly with TESVOLT AG, the "Energy Storage Sensor Technology" group at Fraunhofer HHI will build the sensor-integrated modules with differing health conditions. At the Research Centre for Energy Storage Technologies at TU Clausthal, scientists will develop load profile characterization procedures that can be utilized to test the manufactured modules time-optimized.The processed data will be made available to the AI experts at Intuitive.ai GmbH and EoT Labs GmbH. On this basis, the AI will be programmed while also creating a digital twin. The Institute of Welding Technology and Separating Manufacturing Processes at TU Clausthal and AIM Systems GmbH are responsible for laser processing and automating the extraction of defective cells.