December 16, 2019
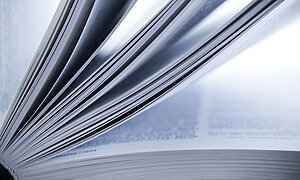
The media group Thomson Reuters has recognized several papers of the Fraunhofer HHI research group Machine Learning. The publication's focus lies on the interpretability of neural networks, the explainability of Artificial Intelligence (AI) and neural net-based quality estimation in images.
Thomson Reuters, a spin-off of the Reuters News Agency and the media company The Thomson Corporation, has published the current "Essential Science Indicators' (ESI) Highly Cited Papers" list of the most cited papers. The "highly cited" award recognizes papers from various subject areas that are among the best one percent of the most frequently cited scientific publications. In the research area "Engineering", four papers from Fraunhofer HHI researchers received this award.
Sebastian Bach, Alexander Binder, Grégoire Montavon, Frederick Klauschen, Klaus-Robert Müller, and Wojciech Samek: On Pixel-wise Explanations for Non-Linear Classifier Decisions by Layer-wise Relevance Propagation
Machine Learning methods solve a variety of tasks very successfully. However, in most cases they have the disadvantage of not providing information on what led to a particular decision. This paper proposes a general solution to this problem of understanding classification decisions. It uses pixel-by-pixel decomposition of nonlinear classifications and evaluates the procedure in different scenarios.
Grégoire Montavon, Sebastian Lapuschkin, Alexander Binder, Wojciech Samek, and Klaus-Robert Müller: Explaining Nonlinear Classification Decisions with Deep Taylor Decomposition
Nonlinear methods such as Deep Neural Networks (DNNs) are the best option for various demanding Machine Learning problems such as image recognition. Although these methods work impressively well, they have considerable disadvantages: The lack of transparency, the limitation of the interpretability of the solution and thus the area of application in actual practice. The paper presents a new method for the interpretation of generic, multi-layered neural networks. It decomposes the decision on the classification of networks into contributions of their input elements.
Sebastian Bosse, Dominique Maniry, Klaus-Robert Müller, Thomas Wiegand, and Wojciech Samek: Deep Neural Networks for No-Reference and Full-Reference Image Quality Assessment
The paper presents a deep neural network-based approach to image quality assessment (IQA). The network consists of ten convolutional layers and five pooling layers for feature extraction and two fully interconnected layers for regression.
Grégoire Montavon, Wojciech Samek, and Klaus-Robert Müller: Methods for Interpreting and Understanding Deep Neural Networks
The paper addresses the problem of interpreting a model of a deep neural network and explaining its predictions. It is based on a tutorial held at ICASSP 2017. The paper discusses interpretability, technical challenges and application possibilities. Another subject of the work is the "layer-wise relevance" propagation (LRP) technique.
In addition to the "high cited" recognition, this work received the "hot paper" award. This award goes to papers that have been published in the past two years and are then cited immediately. The paper of the Fraunhofer HHI researchers was cited so frequently during a two-month period that it is among the first 0.1 percent compared to other work in the same research area.
The awards "highly cited" and "hot paper" are regarded as indicators for scientifically outstanding papers.