February 18, 2019
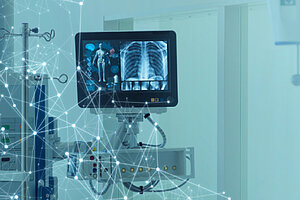
The Machine Learning group at Fraunhofer HHI, which is headed by Wojciech Samek, is advancing the science of Artificial Intelligence (AI) and forging its implementation in uncharted areas such as healthcare and engineering.
Over the past several years, AI has discretely integrated itself into our quotidian lives, contributing to many sectors (e.g., manufacturing, advertizing, and communications). This growing presence of AI is possible because of the availability of big data, increase in computational power, and improvement of machine learning algorithms. However, the potential of AI—that is, how it can be implemented to best serve society—has not yet been realized. This is particularly apparent in the healthcare sector, where AI could provide much-needed relief to healthcare practitioners but there is a hesitancy for adoption. Similarly, in the field of engineering, AI has shown promise in areas like autonomous driving. However, autonomous cars on the streets are a rare sighting. According to Wojciech Samek, this can be ascribed to “the lack of transparency and reliability in AI-based predictions.” Although AI has been shown to outperform humans in certain tasks, its acceptance and adoption, particularly when it is not clear whether the technology is compatible with existing standards of reliability, takes time.
The Machine Learning group is easing the bottleneck of AI: contributing to the explainability, trustworthiness, and privacy preservation of deep model usage, so that AI can be implemented with confidence in all fields—including healthcare and engineering.
Explainable AI
One classic application of machine learning is forming predictions from input data. In the context of healthcare, this can involve image recognition or time series analysis. In the former, imagery (e.g., X-rays) are input into an AI system and a prediction (e.g., diagnosis) is provided as output. For the latter, a time series of data (e.g., ECG) is input into an AI system and a prediction (e.g., diagnosis) is provided as output. If correct, these predictions can revolutionize the workflow of healthcare practitioners—allowing them to focus on the handling and treatment of patients. Similarly, in the field of autonomous driving, image recognition (e.g., identifying street signs) can guide navigation and provide traffic safety.
However, the prediction from an AI system depends on the quality of input data. If these input data are biased or incomplete, errors can be introduced to the AI so that the prediction (e.g., a diagnosis) is unreliable. Therefore, the Machine Learning group is addressing both the quality of input data and explainability of the prediction.
Through involvement in the Berlin Big Data Center and the Berlin Center for Machine Learning, Fraunhofer HHI is resolving data quality issues including small or incomplete data sets and multimodality. To address issues in the quality of healthcare-related imagery (e.g., high dimensionality, spatio-temporal correlations, missing measurements, and relatively small sample sizes), the Machine Learning group is developing new deep learning–based frameworks.
In response to the lack of transparency in AI systems, the Machine Learning group thought outside the (black) box. Through a collaboration with the Technical University of Berlin, they developed a novel method for explainable AI (XAI) called Layer-Wise Relevance Propagation, which enables users to pass backwards through the complex nonlinear neural networks of an AI algorithm, revealing the features leading to prediction (e.g., an image classification or diagnosis). How does this look in practice? For image recognition, XAI can quantify the contribution of each pixel toward a classification. So, if an image of tissue is input into an AI system, the prediction would be the classification (i.e., “cancerous” or “non-cancerous”) plus the basis for that classification (i.e., a heatmap that highlights the pixels leading to the classification). In AI applications for which there is no acceptable margin of error (e.g., healthcare diagnostics or autonomous driving), XAI provides the necessary confidence in AI predictions it also provides an indication of where or how an AI can be improved.
Trustworthy AI
In addition to addressing explainability, the Machine Learning group is providing trust in AI. Deep models can be fooled; this can occur accidentally or as the result of an adversarial attack. In a medical context, changes in the way that data are collected (e.g., replacement of an old with a new X-ray machine) can impact the quality and distribution of input data. Unless the data are properly calibrated, the prediction from an AI algorithm may be untrustworthy. To address this issue, the Machine Learning group is developing techniques to integrate a priori knowledge to constrain the AI-based predictions. In addition, they can provide a measure of confidence.
In the case of an adversarial attack, the input data are modified in a way that is not detectable by humans but still impacts the prediction from an AI algorithm. In the example of autonomous driving, traffic sign manipulation can fool an AI with dire consequences. For sensitive applications, adversarial attacks pose a serious risk and can hinder acceptance of AI technology. The Machine Learning group is exploring advanced denoising techniques to remove the artificial signal in the input data.
Privacy-Preserving AI
Another concern with the use of AI is preserving the privacy of personal data (e.g., health data). Traditionally, health data are moved from the site of collection (i.e., hospital), anonymized, and centralized so that they can train an AI. During this process, there is the risk of data being compromised. To ensure that data are handled with the utmost sensitivity and discretion (e.g., following the requirements of GDPR), and to avoid this type of problem, Wojciech Samek explains that it is possible to “bring the AI to the hospital.” Through a method known as distributed learning, an AI algorithm can be trained at the site (e.g., hospital) of data collection. This is useful, for instance, if data sets from various clinics are to be used to train and update the same (shared) AI algorithm. These data no longer need to be anonymized because they never leave the hospital. Wojciech Samek notes that “for certain ailments or diseases that have a broad geographic distribution, this enables us to train an AI model while avoiding the logistical and legal barriers involved with removing data from the collection site.”
These three aspects (explainability, trustworthiness, and preservation of privacy) are a few examples of how the Machine Learning group is advancing the field of AI and permitting its implementation in sensitive fields such as healthcare and engineering. By providing insight into the decisions made by AI, the Machine Learning Group brings us one step closer to the ability to create standards of reliability (and certifications) for AI.