We are bridging the gap between academic research and practical applications of Artificial Intelligence (AI) by addressing fundamentals, quality assurance, and approaches to overcome practical limitations.
Our activities enable AI to be standardized and implemented in multiple sectors.
Competencies at Fraunhofer HHI
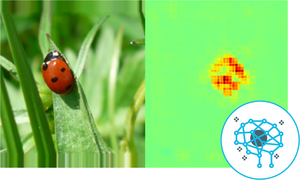
Fundamental Research and Tools
- Deep learning for various data modalities
- Pioneers of explainable AI
- Self-supervised pre-training and transfer learning
- Communication-efficient federated learning
- Neural network compression
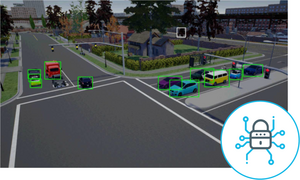
Quality Criteria for AI
- Generalizability:
correct predictions on unseen data - Explainability:
allows for verification and new insights - Robustness:
resistance to attacks and manipulations - Uncertainty:
learn about what the AI does not know - AI Auditing:
assess the quality of your AI
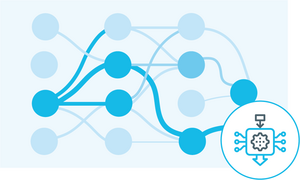
Applications in Frontier Domains
- Medicine:
from ECG to fMRI - Sciences (Bioinformatics, Physics, Biology):
from proteins to moving cells - Environment:
from reducing air pollution to forecasting extreme weather events - Communications:
from video compression to 5G - Autonomous Driving:
from object detection to self-driving vehicles
Standardization
- ITU/WHO Focus Group on “AI for Health”
- ITU/WMO/UNEP Focus Group on “AI for Natural Disaster Management”
- ISO/IEC-MPEG Standards on Neural Network Representation (NNR)
- Further standardization activities:
ISO SC42 (international), DIN NA 043 (national)