The introduction of wireless networks for mobile communication made their optimization a central issue due to the ever-increasing demand. With current wireless networks such as 5G, the key parameters are not only the data rate and capacity, but also requirements regarding energy efficiency, latency, eavesdropping security and others. Thus, enormous amounts of data can accumulate which can only be advantageously processed by means of Machine Learning.
The mobile terminals collect the major part of the data. Therefore, they use their transmission and additionally recorded environmental data to gain a more comprehensive overview of the mobile communication cell supplying them. Using this cognitively acquired additional knowledge, it becomes possible to act with some foresight. Further information, e.g. from the Internet, can be used to support this. For example, the automatic evaluation of an event calendar can provide the location and time of major events, which can then be taken into account in advance when optimizing a wireless network.
The long-term goal is not only to enable the individual mobile user to gain cognitive abilities with regard its immediate environment, but also to combine and further process the cognitive knowledge of all mobile users in such a way that cognitive mobile communications management is achieved, i.e. that the wireless network can control itself largely autonomously.
In order to achieve this goal, the department Wireless Communications and Networks develops, investigates and tests various methods for the optimization of wireless networks. One goal is, for example, to produce detailed maps of the entire wireless network for various relevant parameters, which can then be evaluated to achieve the best possible condition for the wireless network. Relevant parameters are, for example, the traffic density or the capacity of a network, whose relationships, including the input data used for this purpose, are shown as examples in the following figure.
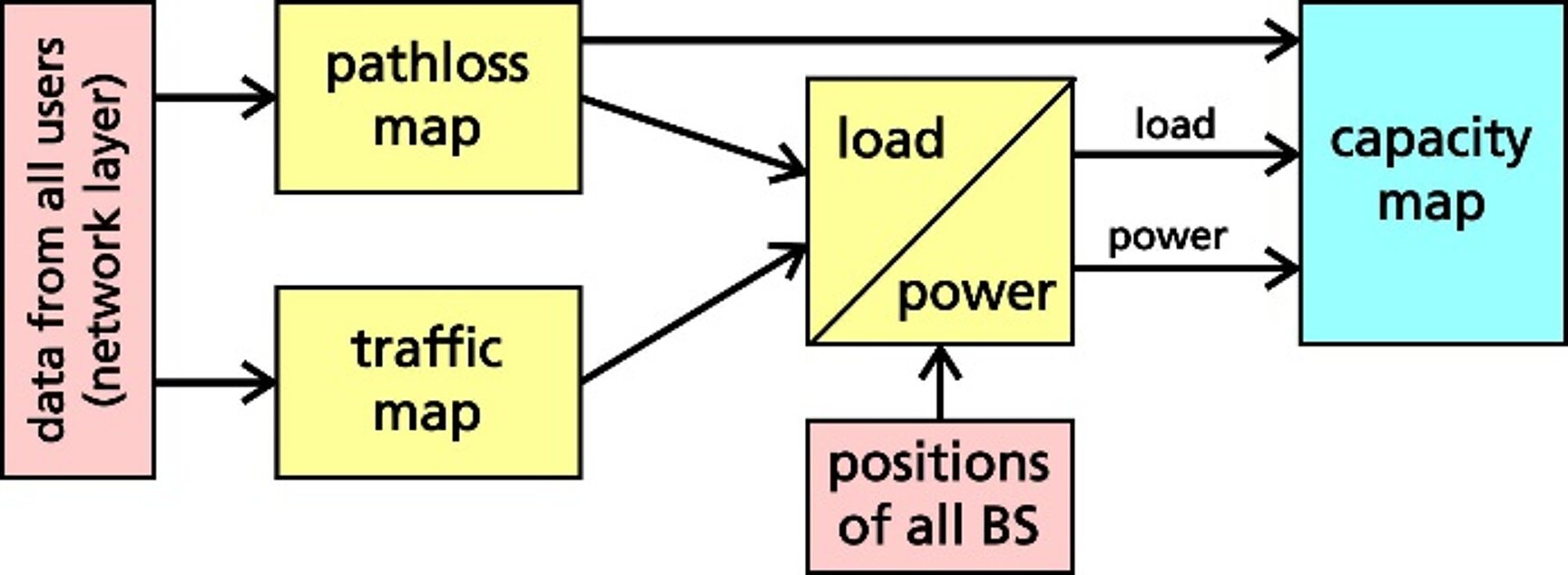
Examples of topics dealt with in the department of Wireless Communications and Networks include: prediction of data traffic based on measured data including context-related additional information [1], learning so-called radio maps using measured user data [2], [3], so-called data driven methods for estimating traffic density [4], protocol questions in very dense and interfering networks [5], various approaches for determining traffic density and capacity distribution [6], [7], [8], [9], [10], measurement of interferences to detect anomalies [11] and questions of energy efficiency [12], [13].
References
[1] R. L. G. Cavalcante, S. Stańczak, M. Schubert, A. Eisenblätter, and U. Türke, "Toward Energy-Efficient 5G Wireless Communication Technologies," IEEE Signal Processing Magazine, vol. 31, no. 6, pp. 24-34, Nov. 2014
[2] M. Kasparick, R. L. G. Cavalcante, S. Valentin, S. Stańczak, and M. Yukawa, "Kernel-Based Adaptive Online Reconstruction of Coverage Maps with Side Information," IEEE Transactions on Vehicular Technology, vol. 65, no. 7, pp. 5461-5473, July 2016
[3] K. Oltmann, R. L. G. Cavalcante, S. Stańczak, and M. Kasparick, "Interference Identification in Cellular Networks via Adaptive Projected Subgradient Methods," in Proc. IEEE Asilomar Conference on Signals, Systems, and Computers, Nov. 2013
[4] D. A. Awan, R. L. G. Cavalcante, and S. Stańczak, "A robust machine learning method for cell-load approximation in wireless networks," in Proc. IEEE International Conference on Acoustics, Speech and Signal Processing (ICASSP), Apr. 2018
[5] N. Agrawal, M. Frey and S. Stanczak, A Scalable Max-Consensus Protocol For Noisy Ultra-Dense Networks. IEEE 20th International Workshop on Signal Processing Advances in Wireless Communications (SPAWC), July 2019
[6] R. L. G. Cavalcante and S. Stańczak, "The role of asymptotic functions in network optimization and feasibility studies," in Proc. IEEE Global Conference on Signal and Information Processing (GlobalSIP), Nov. 2017
[7] R. L. G. Cavalcante and S. Stańczak, "Peak load minimization in load coupled interference networks," in Proc. IEEE International Conference on Acoustics, Speech and Signal Processing (ICASSP), Mar. 2017
[8] R. L. G. Cavalcante, M. Kasparick, and S. Stańczak, "Max-min utility optimization in load coupled interference networks," IEEE Trans. Wireless Comm., vol. 16, no. 2, pp. 705-716, Feb. 2017
[9] R. L. G. Cavalcante, S. Stańczak, J. Zhang, and H. Zhuang, "Low Complexity Iterative Algorithms for Power Estimation in Ultra-Dense Load Coupled Networks," IEEE Trans. Signal Processing, vol. 64, no. 22, pp. 6058-6070, Nov. 2016
[10] R. L. G. Cavalcante, Y. Shen, S. Stańczak, "Elementary Properties of Positive Concave Mappings with Applications to Network Planning and Optimization," IEEE Trans. Signal Processing, vol. 64, no. 7, pp. 1774-1783, April 2016
[11] K. Ralinovski, M. Goldenbaum and S. Stanczak, Energy-efficient Classification for Anomaly Detection: The Wireless Channel as a Helper, IEEE ICC, 2016
[12] E. Pollakis, R. L. G. Cavalcante, and S. Stańczak, "Base station selection for energy efficient network operation with the majorization-minimization algorithm," in SPAWC June 2012
[13] E. Pollakis, R. L. G. Cavalcante, and S. Stańczak, "Traffic Demand-Aware Topology Control for Enhanced Energy-Efficiency of Cellular Networks," EURASIP Journal on Wireless Communications and Networks, vol. 2016, no. 1, pp. 1-17, 2016