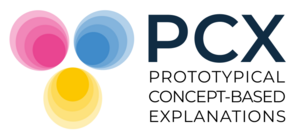
Prototypical Concept-based Explanations
Concept-based explanations improve our understanding of deep neural networks by communicating the driving factors of a prediction in terms of human-understandable concepts. However, investigating each and every sample for understanding the model behavior on the whole dataset can be a laborious task. With PCX (Prototypical Concept-based eXplanations), we introduce a novel explainability method that enables a quicker and easier understanding of model (sub-)strategies on the whole dataset by summarizing similar concept-based explanations into prototypes.
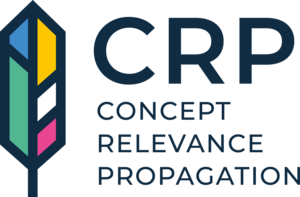
Concept Relevance Propagation
CRP is a cutting-edge explainability method for deep neural networks that allows to understand AI predictions on the concept level. As an advancement of LRP, CRP indicates not only which input features are relevant, but also communicates which concepts are used, where they are located in the input and which parts of the neural network are responsible. Thus, CRP sets new standards in AI validation and interaction with a high level of human-understandability.

Auditing and Certification of AI Systems
The Fraunhofer Heinrich Hertz Institute (HHI), together with TÜV Association and the Federal Office for Information Security (BSI) have published the jointly developed whitepaper entitled "Towards Auditable AI Systems: From Principles to Practice" which proposes to employ a newly developed “Certification Readiness Matrix” (CRM) and presents its initial concept.
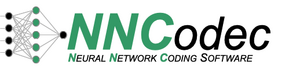
Fraunhofer Neural Network Encoder/Decoder (NNCodec)
For efficient neural network coding, Fraunhofer HHI has developed an easy-to-use and NNC standard conform software (NNCodec). The source code is available on GitHub.

Layer-wise Relevance Propagation (LRP)
Layer-wise Relevance Propagation (LRP) is a patented technology for explaining predictions from deep neural networks and other "black box" models. The explanations produced by LRP (so-called heatmaps) allow the user to validate the predictions of the AI model and to identify potential failure modes.
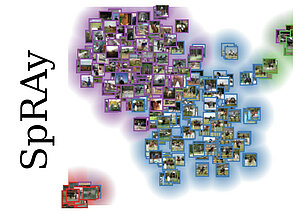
Spectral Relevance Analysis (SpRAy)
XAI methods such as LRP aim to make the prediction of ML models transparent by providing interpretable feedback on individual predictions of the model and by evaluating the importance of input characteristics in relation to specific samples. Based on these individual explanations, SpRAy allows to obtain a general understanding of the sensitivities of a model, its learned features and concept codes.
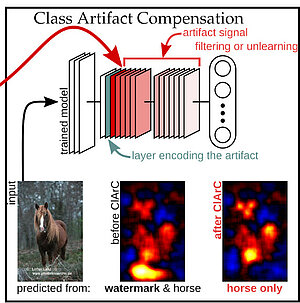
Class Artifact Compensation (ClArC)
Today's AI models are usually trained with extremely large, but not always high-quality, data sets. Undetected errors in the data or incorrect correlations often prevent the predictor from learning a valid and fair strategy for solving the task at hand. The ClArC technology identifies potential errors in the models based on their (LRP) explanations and retrains the AI in a targeted manner in order to solve the problem.