Our research on XAI has seen numerous applications in computer vision (e.g. in image classification1, visual question answering2, image captioning3, video analysis4), as well as in time series predictions (speech processing5, reinforcement learning6, 7, 8) and natural language processing (text classification9, sentiment analysis10). Besides, our XAI methods were employed to analyze various biomedical, medical and scientific records (EEG11, EMG12, fMRI13, human gait analysis14, histopathology15, therapy prediction16, toxicology17, protein-ligand scoring18, meteorology19).
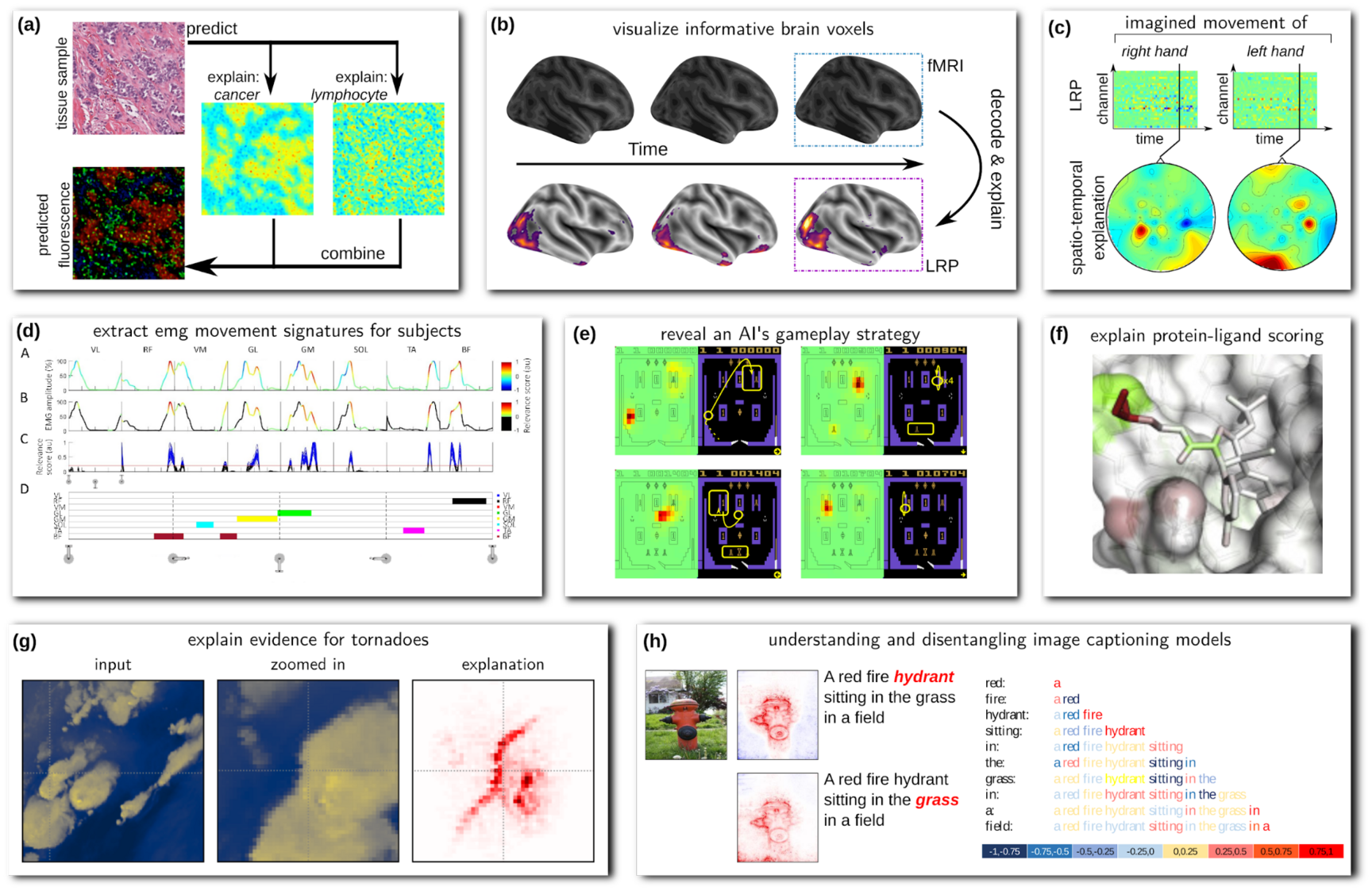
In all these domains and applications, our explanations were successfully able to highlight determinant aspects of the model's prediction for single data points, but also global characteristics of the model's behavior over the entire dataset, and thus verify model behaviour, e.g., on the grounds of previous scientific knowledge, and demonstrate that several of the analyzed machine learning approaches have been able to learn and recognize complex (e.g. chemical or biological) interactions and patterns.
Publications
- Miriam Hägele, Philipp Seegerer, Sebastian Lapuschkin, Michael Bockmayr, Wojciech Samek, Frederick Klauschen, Klaus-Robert Müller, Alexander Binder (2020):
Resolving Challanges in Deep Learning-Based Analyses of Histopathological Images using Explanation Methods,
Scientific Reports, Nature Research, vol. 10, Springer Nature, p. 6423, DOI: 10.1038/s41598-020-62724-2, April 2020 - Fabian Horst, Sebastian Lapuschkin, Wojciech Samek, Klaus-Robert Müller, Wolfgang I. Schöllhorn (2019):
Explaining the Unique Nature of Individual Gait Patterns with Deep Learning,
Scientific Reports, Nature Research, vol. 9, London, UK, Springer Nature, p. 2391, DOI: 10.1038/s41598-019-38748-8, February 2019 - Armin W. Thomas, Hauke R. Heekeren, Klaus-Robert Müller, Wojciech Samek (2019):
Analyzing Neuroimaging Data Through Recurrent Deep Learning Models,
Frontiers in Neuroscience, vol. 13, p. 1321, DOI: 10.3389/fnins.2019.01321, December 2019 - Jeroen Aeles, Fabian Horst, Sebastian Lapuschkin, Lilian Lacourpaille, François Hug (2021):
Revealing the unique features of each individual's muscle activation signatures,
Journal Of The Royal Society Interface, vol. 18, no. 174, The Royal Society, p. 20200770, ISSN: 1742-5662, DOI: https://doi.org/10.1098/rsif.2020.0770, 13th January 2021 - Irene Sturm, Sebastian Lapuschkin, Wojciech Samek, Klaus-Robert Müller (2016):
Interpretable Deep Neural Networks for Single-Trial EEG Classification,
Journal of Neuroscience Methods, Elsevier Inc., vol. 274, pp. 141-145, DOI: 10.1016/j.jneumeth.2016.10.008, December 2016 - Sebastian Lapuschkin, Stephan Wäldchen, Alexander Binder, Grégoire Montavon, Wojciech Samek, Klaus-Robert Müller (2019):
Unmasking Clever Hans Predictors and Assessing What Machines Really Learn,
Nature Communications, vol. 10, London, UK, Nature Research, p. 1096, DOI: 10.1038/s41467-019-08987-4, March 2019 - Leila Arras, José Arjona-Medina, Michael Widrich, Grégoire Montavon, Michael Gillhofer, Klaus-Robert Müller, Sepp Hochreiter, Wojciech Samek (2019):
Explaining and Interpreting LSTMs,
In: Lars Kai Hansen, Grégoire Montavon, Klaus-Robert Müller, Wojciech Samek, Andrea Vedaldi, Explainable AI: Interpreting, Explaining and Visualizing Deep Learning (Lecture Notes in Computer Science) , pp. 211-238, Springer International Publishing, Springer Nature Switzerland, vol. 11700, DOI: 10.1007/978-3-030-28954-6_11, August 2019 - Vignesh Srinivasan, Sebastian Lapuschkin, Cornelius Hellge, Klaus-Robert Müller, Wojciech Samek (2017):
Interpretable human action recognition in compressed domain,
New Orleans, Louisiana, USA, Proceedings of the IEEE International Conference on Acoustics, Speech and Signal Processing (ICASSP 2017), LA, paper, pp. 1692-1696, DOI: 10.1109/icassp.2017.7952445, March 2017 - Seul-Ki Yeom, Philipp Seegerer, Sebastian Lapuschkin, Alexander Binder, Simon Wiedemann, Klaus-Robert Müller, Wojciech Samek (2021):
Pruning by Explaining: A Novel Criterion for Deep Neural Network Pruning,
Pattern Recognition, vol. 115, page 107899, DOI: doi.org/10.1016/j.patcog.2021.107899