Hyperspectral imaging records images of very many, closely spaced wavelengths, ranging from wavelengths in the ultraviolet to the long-wave infrared. Reflectance characteristics can be used to derive information in the different wavelengths can be used to derive information on materials in industrial imaging, vegetation and plant health status in agriculture, or tissue characteristics in medicine. In contrast to a panchromatic image, usually, a lower spatial resolution is obtained. There are different techniques for acquiring hyperspectral data, illustrated as slices through the hyperspectral data cube. We develop capturing, calibration and data analysis techniques for HSI imaging.
Setups
We investigate different setup:
- Hyperspectral light-field setup: We use a hyperspectral light field camera, which introduces the spectral dimension to the typical light field imaging. Every single subimage records a slightly different wavelength of the scene while conserving all other positive aspects of the light field. This allows us to capture the scene in the spectral range of 350-1000nm including 3D information with frame rates of above 1fps.
- Stereo-Snapshot-Mosaic setup: We use two snapshot mosaic cameras holding different sensors. One camera holds a 4×4- and the other a 5×5-mosaic pattern, which includes 16 and 25 wavelength bands, respectively. The 4×4-mosaic sensor is sensitive in the visible (VIS) spectrum while the 5×5-mosaic sensor is sensitive in the near-infrared (NIR) spectrum. Thus, each pixel in the mosaic pattern corresponds to one wavelength band. Both cameras are set up in a stereoscopic way to acquire depth information in addition to the 41 spectral bands and the spatial resolution.
- Filter-wheel setup: For medical applications, we built an imaging setup based on a prototype of the digital surgical microscope ARRISCOPE including a digital camera featuring a monochrome CMOS sensor as recording device and a Xenon illumination unit. In front of the illumination unit, a filter wheel selects a specific wavelength band that reaches the tissue. This approach allows a scanning of 19 spectral bands in the range of 400nm to 850nm in steps of 20nm from 400nm to 700nm and 50nm from 700nm to 850nm. The 20th channel only holds a UV-filter and allows full intensity capturing.
- Flash-Sequence setup: Also for medical applications, we use a digital surgical microscope with an RGB sensor and an LED light source. The multispectral analysis unit uses the integrated LEDs synchronized to the RGB sensor frequency capturing a sequence of four consecutive different illuminated images. The spectral response of the three RGB channels captures the four different illuminated images. Hence, twelve spectral images can be recorded by using the LED sequence combined with the RGB channels. Each spectral image captures information about specific spectral characteristics, which recur differently in different spectra.
Calibration
The usage of multi- and hyperspectral imaging modalities requires a robust calibration with reference data due to different impacts and challenges that comes with spectral capturing. Since the measurement of the reference data includes uncertainties, errors will propagate into the corrected data, which cannot be quantified in the postprocessing. In order to make the acquired data comparable between the different setups and other spectral acquisition techniques, a joint calibration chain is developed.
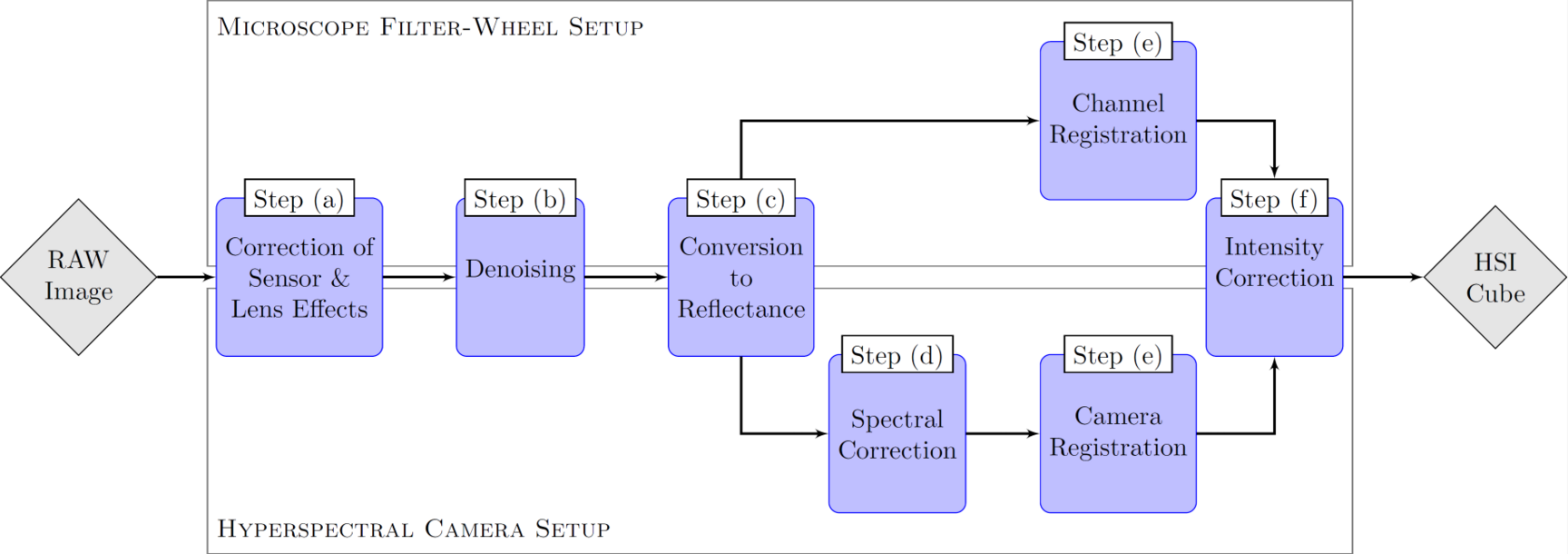
Demosaicing with Ground Truth Data
Single-camera-one-shot (mosaic snapshot) techniques allow multiple spectral bands to be captured simultaneously in a simple and compact system. However, a mosaic pattern is always a compromise between spatial and spectral resolution, as with increasing mosaic size (for higher spectral resolution) the spatial resolution decreases. This spectral-spatial trade-off can be resolved through interpolation or prediction of the missing spectral values, such that the final HSI exhibits higher resolution (spatial and spectral).
We develop different network approaches for individual applications. In all applications, our gosl is to achieve best image quality by applying fast and as small as possible networks. In order to train our network with real ground truth images, we created a customized dataset from publicly available as well as new self-recorded ground truth data. A HSI captured with a 4×4 mosaic pattern contains information for 1 out of 16 wavelength bands in each pixel only. For training the demosaicing algorithm, ground-truth data is necessary such that for each pixel, full spectral information exists across all 16 wavelength bands. Hence, we captured an unprecedented new HSI dataset, providing accurate ground truth upsampling information. We capture in a controlled environment with a 4×4 snapshot camera. For the generation of the ground-truth information, we captured 16 images with precise pixel-wise shifting, meandering along the 4 × 4 mosaic pattern for each scene, using a computer-controlled shifting unit. To achieve accurate 1-pixel shifts, the camera was calibrated and the scene-camera distance was measured. After acquiring the 4 × 4 = 16 images, a full resolution image with 16 wavelengths was created by resampling the image stack. At each position, we captured and averaged several images to decrease the influence of sensor noise. In order to account for illumination differences and work with reflectance intensity, all captured images were corrected using the above described calibration pipeline.
Spectral Analysis
We develop techniques to visualize the extracted hyperspectral information appropriately on a display or in a microscope and endoscope, e.g. by enhancing the reconstructed data in the relevant spectral range. We are able to calculate and visualize RGB images, physiological coefficients as well as spectral parameter for object analysis and differentiation. A distinguished visualization allows the intraoperative usage of these additional information.
Our work on spectral analysis takes place across different topics. Thereby, the focus is on two complexes: agriculture and medicine. Different neural networks will be developed to analyze plant physiological traits using data from different sensors (e.g. drone, close-up). The hyperspectral data and features are fused with further information to obtain information on plant identification, diseases, stress, nitrogen demand, growth.
For the medical image analysis, tissue differentiation as well as the analysis of physiological parameter is of highest interest. Tissue differentiation can be performed by enhancing specific spectral regions to highlight specific tissues, e.g. 460 nm to 480 nm for nerves. Further, different neural networks are trained to allow a cross-sectional tissue analysis and differentiation. In addition, different physiological parameter like water content, oxygenation and perfusion can be analyzed. For intraoperative usage, a reasonable visualization of the information extracted from the hyperspectral data is needed. A classical RGB image can be calculated from the hyperspectral data using the CIE color matching functions. Then, enhanced image data are added to the blue channel of the calculated RGB image or can be presented using heat maps.
Publications
E. L. Wisotzky, A. Hilsmann, P. Eisert
3D Hyperspectral Light-Field Imaging: a first intraoperative implementation,
Current Directions in Biomedical Engineering, 9(1):611-314, 2023 doi: https://doi.org/10.1515/cdbme-2023-1153
E. L. Wisotzky, J. Triller, B. Kossack, B. Globke, P. Arens, A. Hilsmann, P. Eisert
From Multispectral-Stereo to Intraoperative Hyperspectral Imaging: a Feasibility Study,
Current Directions in Biomedical Engineering, 9(1):311-314, 2023 doi: https://doi.org/10.1515/cdbme-2023-1078
E. L. Wisotzky, C. Daudkane, A. Hilsmann, P. Eisert
Hyperspectral Demosaicing of Snapshot Camera Images Using Deep Learning,
DAGM GCPR 2022: Pattern Recognition, pp. 198-212, September 2022, doi: https://doi.org/10.1007/978-3-031-16788-1_13
E. L. Wisotzky, F. C. Uecker, J.-C. Rosenthal, P. Arens, A. Schneider
Near-UV to near-IR multispectral illumination in a digital microscope,
Current Directions in Biomedical Engineering, 7(2):464-467, 2021, doi: https://doi.org/10.1515/bmt-2021-6036
E. L. Wisotzky, B. Kossack, F. C. Uecker, P. Arens, A. Hilsmann, P. Eisert
Validation of two techniques for intraoperative hyperspectral human tissue determination,
Journal of Medical Imaging, 7(6):065001, 2020. [pdf]
E. L. Wisotzky, Jean-Claude Rosenthal, Ulla Wege, Anna Hilsmann, Peter Eisert, Florian Uecker
Surgical Guidance for Removal of Cholesteatoma Using a Multispectral 3D-Endoscope,
Sensors, 20(18):5334, September 2020, doi: 10.3390/s20185334
E. L. Wisotzky, P. Arens, S. Dommerich, A. Hilsmann, P. Eisert, F. C. Uecker,
Determination of optical properties of cholesteatoma in the spectral range of 250 to 800 nm, Biomedical Optics Express, 11(3):1489, 2020. [pdf]
E. L. Wisotzky, J.-C. Rosenthal, F. C. Uecker, A. Hilsmann, P. Eisert,
A Multispectral 3D-Endoscope for Cholesteatoma Removal,
Current Directions in Biomedical Engineering, 6(3):20203065, 2020. [pdf]
E. L. Wisotzky, F. C. Uecker, S. Dommerich, A. Hilsmann, P. Eisert, P. Arens,
Determination of optical properties of human tissues obtained from parotidectomy in the spectral range of 250 to 800 nm, Journal of Biomedical Optics, 24(12):125001, 2019.
E. L. Wisotzky, B. Kossack, F. C. Uecker, P. Arens, S. Dommerich, A. Hilsmann, P. Eisert,
Validation of two techniques for intraoperative hyperspectral human tissue determination, Proceedings of SPIE, 10951:109511Z, 2019.
E. L. Wisotzky, F. C. Uecker, P. Arens, S. Dommerich, A. Hilsmann, and P. Eisert,
Intraoperative hyperspectral determination of human tissue properties, Journal of Biomedical Optics, 23(9):091409, 2018.
E. L. Wisotzky,
HSI in der diagnostischen und therapeutischen Medizin,
In M. Sackewitz (Hrsg.), Leitfaden zur Hyperspektralen Bildgebung, Vision Leitfaden 19, S. 80-83, Stuttgart, Fraunhofer Verlag, 2019. ISBN 978-3-8396-1502-7 [pdf]