With the availability of large databases and recent improvements in deep learning methodology, the performance of AI systems is reaching or even exceeding the human level on an increasing number of complex tasks. Deep Neural Networks (DNNs) are particularly successful, because they are forming an efficient internal representation of the learning problem by applying a sequence of nonlinear transformations to the input. This provides a very efficient way to fight the curse of dimensionality and learn the optimal features for a given problem.
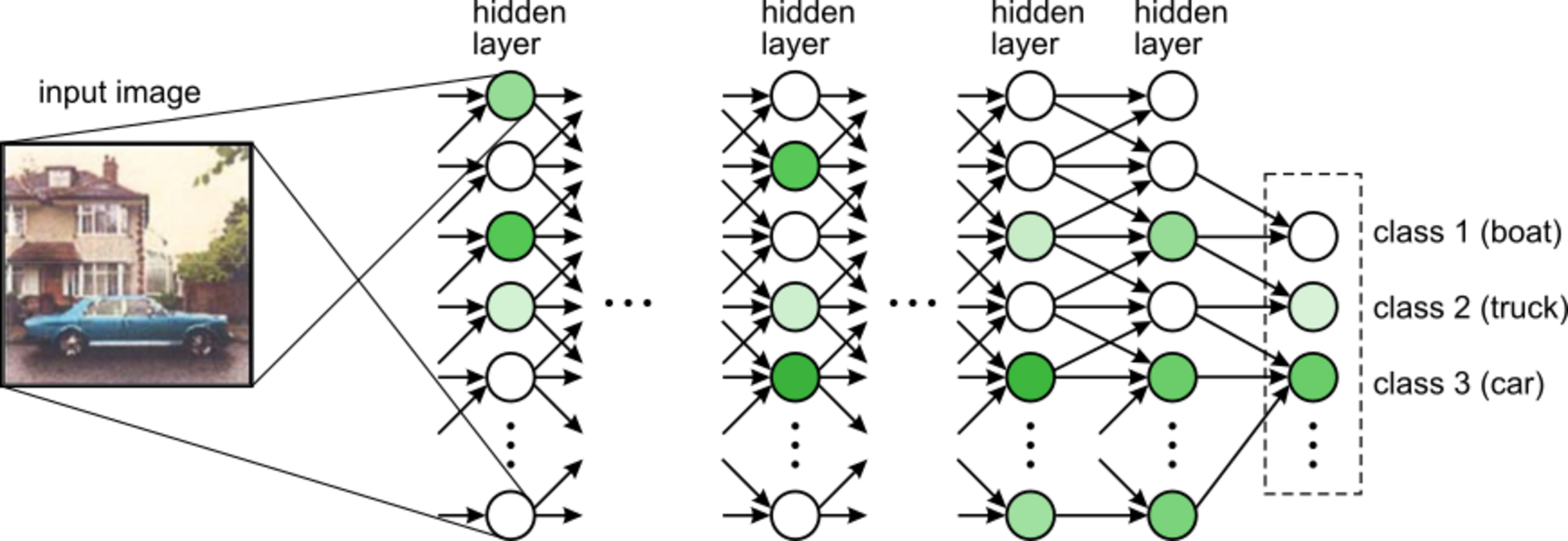
We explore novel deep architectures for various classification and recognition tasks on image, text and video data, including image classification, compressed domain object tracking, document topic classification, sentiment analysis, visual question answering, human action recognition and detection of face morphing attacks.
The main focus of our work lies on
- Information-theoretical analysis of deep neural networks.
- Application of deep learning to various problems.
- Development of novel convolutional and recurrent architectures.
- Understanding and interpreting the reasoning of the system.
- Incorporation of a priori knowledge and side information into the learning process.
Publications
- S. Bosse, D. Maniry, K.-R. Müller, T. Wiegand, and W. Samek, "Deep Neural Networks for No-Reference and Full-Reference Image Quality Assessment", IEEE Transactions on Image Processing, September 2017.
- L. Arras, F. Horn, Grégoire Montavon, K.-R. Müller, and W. Samek, "What is Relevant in a Text Document?": An Interpretable Machine Learning Approach" PLOS ONE, vol. 12, no. 8, pp. e0181142, August 2017.
- C. Seibold, W. Samek, A. Hilsmann, and P Eisert, "Detection of Face Morphing Attacks by Deep Learning", Proceedings of the 16th International Workshop on Digital Forensics and Watermarking (IWDW), Magdeburg, Germany, pp. 107-120, August 2017.
- A. Marban, V. Srinivasan, W. Samek, J. Fernández, and A. Casals, "Estimating Position & Velocity in 3D Space from Monocular Video Sequences using a Deep Neural Network", Proceedings of the ICCV'17 Workshop on Assistive Computer Vision and Robotics (ACVR), Venice, Italy, October 2017.
- S. Lapuschkin, A. Binder, K.-R. Müller, and W. Samek, "Understanding and Comparing Deep Neural Networks for Age and Gender Classification", Proceedings of the ICCV'17 Workshop on Analysis and Modeling of Faces and Gestures (AMFG), Venice, Italy, October 2017.
- S. Lapuschkin, A. Binder, G. Montavon, K.-R. Müller, and W. Samek, "Analyzing Classifiers: Fisher Vectors and Deep Neural Networks", Proceedings of the IEEE Conference on Computer Vision and Pattern Recognition (CVPR), Las Vegas, NV, USA, pp. 2912-20, June 2016.
- F. Arbabzadeh, G. Montavon, K.-R. Müller, and W. Samek, "Identifying Individual Facial Expressions by Deconstructing a Neural Network", Pattern Recognition - 38th German Conference, GCPR 2016, Lecture Notes in Computer Science, vol. 9796, pp. 344-54, Springer International Publishing, August 2016.
- S. Bach, A. Binder, G. Montavon, F. Klauschen, K.-R. Müller, and W. Samek, “On Pixel-wise Explanations for Non-Linear Classifier Decisions by Layer-wise Relevance Propagation”, PLOS ONE, vol. 10, no. 7, pp. e0130140, July 2015.