Introduction
In cellular communications, the transmission of information is sometimes plagued by problems (e.g., the corruption of data). In response, receivers request a retransmission of information. Hybrid automatic repeat request (HARQ) is a well-established mechanism that combines forward error-correcting coding with automatic repeat request (ARQ) error control.
HARQ has a tradition of use in Long-Term Evolution (LTE) technologies. Now, 5G applies the same mechanism to achieve high spectral efficiency. Whereas in the previous generation (with LTE) the HARQ protocol was limited by many restrictions, 5G allows a fully flexible operation to make maximum use of time and frequency diversity. However, a major drawback of HARQ is the introduction of added latency. This poses an issue for latency-sensitive communication, such as factory automation, self-driving cars, and control for VR streaming.
The Multimedia Communications group explores methods to minimize the added latency, while benefitting from HARQ features such as high reliability and high spectral efficiency. The approach is based on HARQ feedback prediction, which applies various machine learning methods to predict the decodability of a partially received signal. Here, an emphasis is placed on so-called low-density parity-check (LDPC) subcodes, which characterize the features in the partial signal of the original code. By incorporating the code structure into the prediction mechanism, we achieve significant improvement over simple average likelihood estimators such as average log-likelihood ratio (LLR) thresholding.
Predictive HARQ
With Predictive HARQ, we focus on providing the HARQ feedback earlier by predicting the decoding outcome, designated as Early HARQ (E-HARQ) in the following. As will be discussed in detail below, the HARQ RTT is composed of several components including propagation delays and processing delays. Our approach focuses solely on reducing the delay on the receiver side from the start of reception until the feedback is sent. Hence, all other components contributing to the HARQ RTT will be considered fixed for this purpose. Typical E-HARQ schemes provide the feedback on the decodability of the received signal ahead of the end of the actual transmission process. The crucial component in this setting is the classification algorithm that provides the feedback, which we aim to optimize using machine learning techniques.
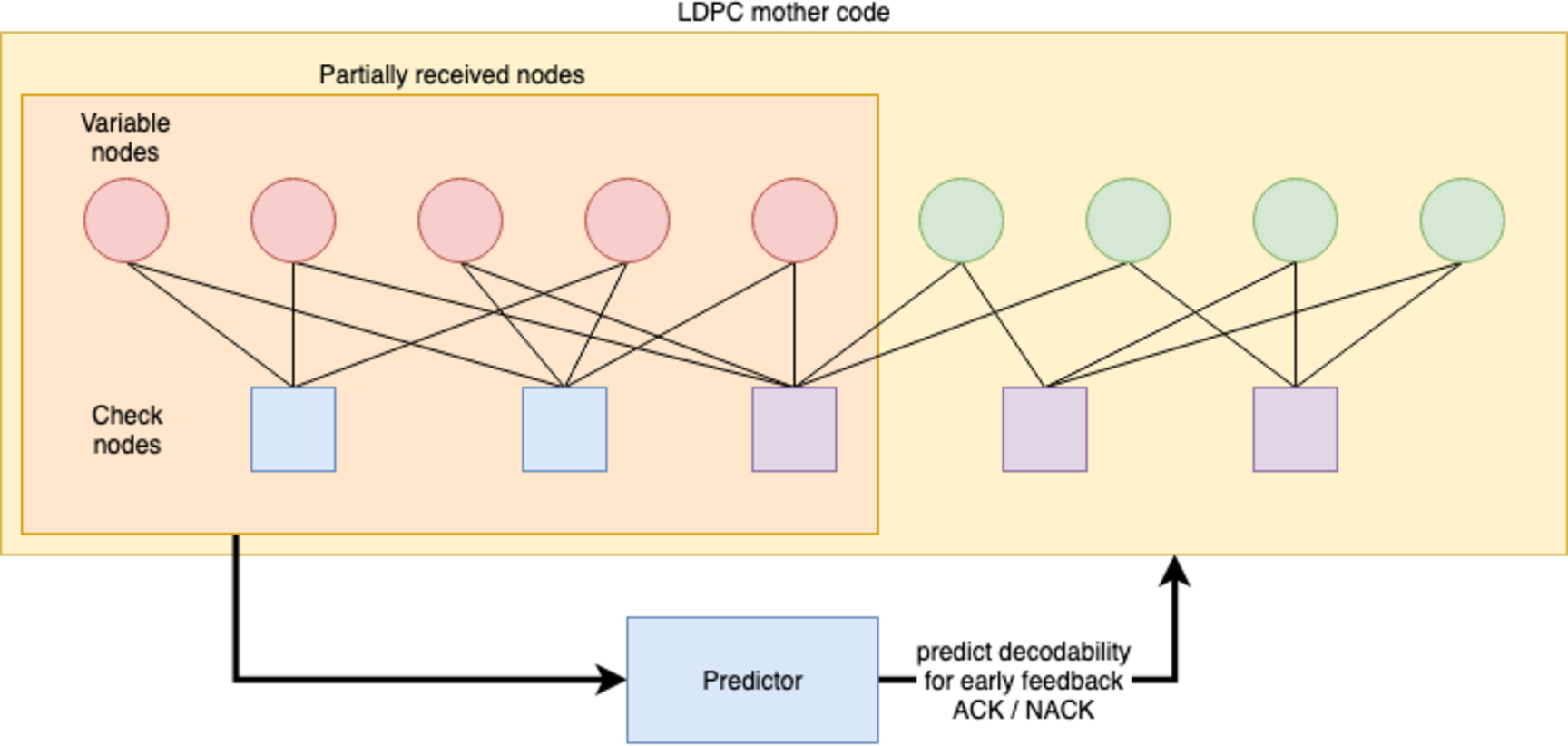
Publications
- N. Strodthoff, B. Göktepe, T. Schierl, C. Hellge, W. Samek, "Enhanced machine learning techniques for early HARQ feedback prediction in 5G", IEEE Journal on Selected Areas in Communications, vol. 37, no. 11, pp. 2573-2587, Nov. 2019. [download]
- B. Göktepe, S. Fähse, L. Thiele, T. Schierl, C. Hellge, "Subcode-based Early HARQ for 5G", Proceedings of IEEE International Conference on Communications Workshops (ICC Workshops), Kansas City, MO, USA, May 2018. [download]
- N. Strodthoff, B. Göktepe, T. Schierl, W. Samek, C. Hellge, "Machine Learning for Early HARQ Feedback Prediction in 5G", 2018 IEEE Globecom Workshops (GC Wkshps), Abu Dhabi, United Arab Emirates, 2018, pp. 1-6. [download]
Standardization
- B. Göktepe, T. Fehrenbach, and C. Hellge (Fraunhofer HHI):R1-1720492, Early HARQ for URLLC, 3GPP TSG-RAN WG1#91, Reno, USA, November 2017. [download]
- B. Göktepe, T. Fehrenbach, and C. Hellge (Fraunhofer HHI): R1-1703309, On HARQ feedback schemes for NR, 3GPP TSG-RAN WG1#88, Athens, Greece, February 2017. [download]
- B. Göktepe, T. Fehrenbach, and C. Hellge (Fraunhofer HHI): R1-1700647, Aggressive early Hybrid ARQ for NR, 3GPP TSG-RAN WG1#87, Spokane, USA, January 2017. [download]