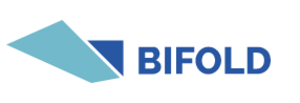
Berlin Institute for the Foundations of Learning and Data
July 2018 – present
BIFOLD research groups conduct fundamental research on a wide range of topics concerning foundations and methods of Artificial Intelligence (AI). This includes the management and processing of distributed and Big Data. As Machine Learning (ML) is one of the main fields for modern AI and the new wave of AI applications, we also focus on a variety of Machine Learning methods such as reinforced and Bayesian Machine Learning as well as unsupervised and recurrent Deep Learning.
Project Partners
- TU Berlin
- HU Berlin
- FU Berlin
- Charité
- Uni Potsdam
- Uni Braunschweig
- Fraunhofer FOKUS
- DFKI
- Zuse Institute
- WIAS
- MDC
- HPI
- MPI
Publications
[1] | Christopher J. Anders, Leander Weber, David Neumann, Wojciech Samek, Klaus-Robert Müller, and Sebastian Lapuschkin. “Finding and removing Clever Hans: Using explanation methods to debug and improve deep models”. In: Information Fusion 77 (2022), pp. 261–295. ISSN: 1566-2535. DOI: https://doi.org/10.1016/j.inffus.2021.07.015. URL: https://www.sciencedirect.com/science/article/pii/S1566253521001573. |
[2] | Lukas Ruff, Jacob Kauffmann, Robert Vandermeulen, Gregoire Montavon, Wojciech Samek, Marius Kloft, Thomas Dietterich, and Klaus-Robert Müller. “A Unifying Review of Deep and Shallow Anomaly Detection”. In: Proceedings of the IEEE PP (Feb. 2021), pp. 1–40. DOI: 10.1109/JPROC.2021.3052449. |
[3] | Wojciech Samek, Gregoire Montavon, Sebastian Lapuschkin, Christopher Anders, and Klaus-Robert Muller. “Explaining Deep Neural Networks and Beyond: A Review of Methods and Applications”. In: Proceedings of the IEEE 109 (Mar. 2021), pp. 247–278. DOI: 10.1109/JPROC.2021.3060483. |
[4] | Jiamei Sun, Sebastian Lapuschkin, Wojciech Samek, and Alexander Binder. “Explain and improve: LRP-inference fine-tuning for image captioning models”. In: Information Fusion 77 (2022), pp. 233–246. ISSN: 1566-2535. DOI: https://doi.org/10.1016/j.inffus.2021.07.008. URL: https://www.sciencedirect.com/science/article/pii/S1566253521001494. |
[5] | Seul-Ki Yeom, Philipp Seegerer, Sebastian Lapuschkin, Alexander Binder, Simon Wiedemann, Klaus-Robert Müller, and Wojciech Samek. “Pruning by explaining: A novel criterion for deep neural network pruning”. In: Pattern Recognition 115 (2021), p. 107899. issn: 0031-3203. doi: doi.org/10.1016/j.patcog.2021.107899. url: www.sciencedirect.com/science/article/pii/S0031320321000868. |