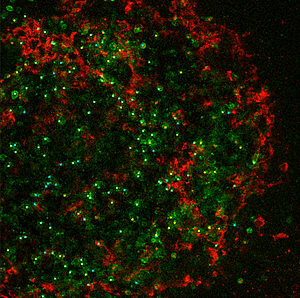
Machine learning-driven multidimensional imaging analysis of reactive and neoplastic lymph nodes
January 2020 – December 2022
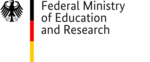
This project was funded by the Federal Ministry of Education and Research on the basis of a decision by the German Bundestag
Combining two-dimensional histological sections, three-dimensional immune-histological sections, and four-dimensional moving lymphocytes, with the aid of machine learning methods (e.g., to address small data sets, incorporate a priori knowledge), Patho234 will produce a realistic human lymph node model consisting of exact tissue and cell structure measurements. This lymph node model will provide insight into inflammatory and neoplastic diseases, both of which impact the immune system in distinct ways. Furthermore, it will be used to build on promising first applications of machine learning methods on histopathology in new (three and four) dimensions.
Project Partners
- Prof. Martin-Leo Hansmann (Frankfurt Institute for Advanced Studies and Goethe University Frankfurt),
- Prof. Frederick Klauschen (Charité University Medicine),
- Prof. Klaus-Robert Müller (Technical University of Berlin)
Publications
[1] | Miriam Hägele, Philipp Seegerer, Sebastian Lapuschkin, Michael Bockmayr, Wojciech Samek, Frederick Klauschen, Klaus-Robert Müller, and Alexander Binder. “Resolving challenges in deep learning-based analyses of histopathological images using explanation methods”. In: Scientific Reports 10.1 (Apr. 2020), p. 6423. ISSN: 2045-2322. DOI: 10.1038/s41598-020-62724-2. URL: https://doi.org/10.1038/s41598-020-62724-2. |
[2] | Wojciech Samek and Klaus-Robert Müller. “Towards Explainable Artificial Intelligence”. In: Explainable AI: Interpreting, Explaining and Visualizing Deep Learning. Ed. by Wojciech Samek, Grégoire Montavon, Andrea Vedaldi, Lars Kai Hansen, and Klaus-Robert Müller. Cham: Springer International Publishing, 2019, pp. 5–22. ISBN: 978-3-030-28954-6. DOI: 10.1007/978-3-030-28954-6_1. URL: https://doi.org/10.1007/978-3-030-28954-6_1. |
[3] | Seul-Ki Yeom, Philipp Seegerer, Sebastian Lapuschkin, Alexander Binder, Simon Wiedemann, Klaus-Robert Müller, and Wojciech Samek. “Pruning by explaining: A novel criterion for deep neural network pruning”. In: Pattern Recognition 115 (2021), p. 107899. ISSN: 0031-3203. DOI: https://doi.org/10.1016/j.patcog.2021.107899. URL: https://www.sciencedirect.com/science/article/pii/S0031320321000868. |