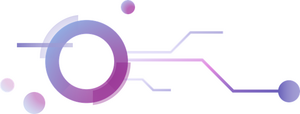
Reconfigurable Superconducting and Photonic Technologies of the Future
March 2023 - February 2027
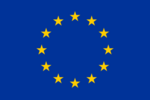
This project has received funding from the European Union’s Horizon Europe research and innovation programme under grant agreement No. 101098717
Computing with light using integrated optics has seen huge progress over the last 3-4 years in multiple fields such as neuromorphic computing, quantum computing, and on-chip data storage. This has created a vast ecosystem that relies on high-speed reconfigurations of nano-photonic circuits (such as their use as synapses or in routing applications) and ultra fast yet high-resolution, low-power photo detection. Currently, it is impossible to combine all these functionalities into an integrated platform that fits onto a single chip.
RESPITE targets a novel platform, which combines vision and cognition on a single chip. This is utilized by using novel superconducting Joule switches as neurons, multi-level phase-change memory elements as synaptic weights, and superconducting single-photon detector arrays as retina. This new platform will allow in-sensor neuromorphic computing with unprecedented performance levels. The platform will have atto-Joule switching power consumption, sub-nanosecond latency, and high compactness (3000 neurons and >100K synapses on <5 mm2). Unlike other superconducting neuromorphic technologies, the new RESPITE platform will be scalable, easy to fabricate, and compatible with low-cost cryostats, high-Tc superconductors, quantum applications, and on-chip learning architectures – making it a game changer for a wide range of users and disciplines.
Within RESPITE, Fraunhofer HHI will develop novel high-performance tiny neural networks (tNNs) adapted to the new platform. Furthermore, for these tNNs, evaluation methods for the new platform will be specified. The tNNs will be developed in 3 stages, with stage 1 only having 16 input, 6 hidden, and 3 output neurons, while stage 3 will have several thousands of neurons. For this, new technologies of value range reduction and prioritized neuron connections for efficient NN architecture sparsification will be developed.
Project Partners
- Single Quantum BV
- Technische Universiteit Delft
- The Chancellor, Masters and Scholars of the University of Oxford
- Universiteit Gent
- Rijksuniversiteit Groningen
- Turkiye Bilimsel ve Teknolojik Arastirma Kurumu
- Fraunhofer Gesellschaft zur Förderung der angewandten Forschung e.V.