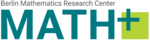
This project was funded by the Berlin Mathematics Research Center (MATH+)
The omnipresence of training data and enhancement of computing capabilities have made deep learning algorithms feasible. Thus far, most deep learning studies have been empirically driven and are usually viewed as "black boxes": they can produce a decision but the grounds for this decision are unclear. In MATH+, a theoretical understanding of the explainability of deep neural networks is developed. For a given decision, the features of input data that played the largest role are identified and the associated uncertainties of a decision are quantified.
Project Partners
- Prof. Gitta Kutyniok (TU Berlin)
- Prof. Klaus-Robert Müller (TU Berlin)
Publications
[1] | Sebastian Bach, Alexander Binder, Grégoire Montavon, Frederick Klauschen, Klaus-Robert Müller, and Wojciech Samek. “On Pixel-Wise Explanations for Non-Linear Classifier Decisions by Layer-Wise Relevance Propagation”. In: PLOS ONE 10.7 (July 2015), pp. 1–46. DOI: 10.1371/journal.pone.0130140. URL: https://doi.org/10.1371/journal.pone.0130140. |
[2] | Tatiana Alessandra Bubba, Gitta Kutyniok, Matti Lassas, Maximilian März, Tatiana Bubba, Wojciech Samek, Samuli Siltanen, and Vignesh Srinivasan. “Learning The Invisible: A Hybrid Deep Learning-Shearlet Framework for Limited Angle Computed Tomography”. In: Inverse Problems (Mar. 2019). DOI: 10.1088/1361-6420/ab10ca. |
[3] | Grégoire Montavon, Sebastian Lapuschkin, Alexander Binder, Wojciech Samek, and Klaus-Robert Müller. “Explaining nonlinear classification decisions with deep Taylor decomposition”. In: Pattern Recognition 65 (2017), pp. 211–222. ISSN: 0031-3203. DOI: https://doi.org/10.1016/j.patcog.2016.11.008. URL: https://www.sciencedirect.com/science/article/pii/S0031320316303582. |
[4] | Grégoire Montavon, Wojciech Samek, and Klaus-Robert Müller. “Methods for interpreting and understanding deep neural networks”. In: Digital Signal Processing 73 (2018), pp. 1–15. ISSN: 1051-2004. DOI: https://doi.org/10.1016/j.dsp.2017.10.011. URL: https://www.sciencedirect.com/science/article/pii/S1051200417302385. |
[5] | Wojciech Samek, Gregoire Montavon, Sebastian Lapuschkin, Christopher Anders, and Klaus-Robert Muller. “Explaining Deep Neural Networks and Beyond: A Review of Methods and Applications”. In: Proceedings of the IEEE 109 (Mar. 2021), pp. 247–278. DOI: 10.1109/JPROC.2021.3060483. |
[6] | Seul-Ki Yeom, Philipp Seegerer, Sebastian Lapuschkin, Alexander Binder, Simon Wiedemann, Klaus-Robert Müller, and Wojciech Samek. “Pruning by explaining: A novel criterion for deep neural network pruning”. In: I 115 (2021), p. 107899. ISSN: 0031-3203. DOI: https://doi.org/10.1016/j.patcog.2021.107899. URL: https://www.sciencedirect.com/science/article/pii/S0031320321000868. |