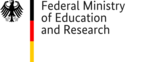
This project was funded by the Federal Ministry of Education and Research on the basis of a decision by the German Bundestag
In this multi-disciplinary project, contributions are made to reliable machine learning (ML). Specifically, methods are explored that can integrate a priori information in machine learning models to enhance their performance (e.g., their reliability and trustworthiness). One possible application of this research is to enhance resistance to adversarial attacks on artificial intelligence.
Project Partners
- FU Berlin
- HU Berlin
- TU Berlin
- University of Potsdam
- Max Planck Institute for the History of Science
- Max Planck Institute for Molecular Genetics
- MDC
- Zuse Institute Berlin
- Charité
- WIAS Berlin
- DHZB
Publications
[1] | Wojciech Samek, Thomas Wiegand, and Klaus-Robert Müller. “Explainable Artificial Intelligence: Understanding, Visualizing and Interpreting Deep Learning Models”. In: ITU Journal: ICT Discoveries - Special Issue 1 - The Impact of Artificial Intelligence (AI) on Communication Networks and Services 1 (Oct. 2017), pp. 1–10. |
[2] | Clemens Seibold, Wojciech Samek, Anna Hilsmann, and Peter Eisert. “Accurate and robust neural networks for face morphing attack detection”. In: Journal of Information Security and Applications 53 (2020), p. 102526. ISSN: 2214-2126. DOI: https://doi.org/10.1016/j.jisa.2020.102526. URL: https://www.sciencedirect.com/science/article/pii/S2214212619302029. |
[3] | Vignesh Srinivasan, Arturo Marban, Klaus-Robert Müller, Wojciech Samek, and Shinichi Nakajima. “Robustifying Models Against Adversarial Attacks by Langevin Dynamics”. In: arXiv e-prints, arXiv:1805.12017 (May 2018), arXiv:1805.12017. arXiv: 1805.12017 [cs.LG]. |