
Content4All
Personalised Content Creation for the Deaf Community in a Connected Digital Single Market
Duration: September 2017 - August 2020
Content4All is a European funded Horizon 2020 project.
Scope of the Project
The project Content4All aims at making content more accessible for the sign language community by implementing an automatic sign-translation workflow with a photo-realistic 3D human avatar. The final result will enable low-cost personalization of content for deaf viewers, without causing disruption for hearing viewers.
Fraunhofer HHI is responsible for the generation and development of a novel hybrid human body model that allows for photo-realistic, real-time rendering and animation. Accordingly, covering all the aspects of model creation, capture, animation, and rendering:
- Exploiting innovative capturing technologies, in order to reproduce fine details (e.g. finger, hands for sign language animation) with limited modeling effort; i.e. fusing rough body models for semantic animation with video samples as dynamic textures.
- Integrating novel rendering methods that combine standard geometry-based computer graphics rendering with warping and blending of video textures.
- Investigating and developing new techniques for realistic and efficient transitions between the video-textures and the geometry motion.
Project Results
Avatar Creation from Multiview-Video Data
In order to create realistic avatars, we captured two human signer at HHI’s volumetric capture-studio, which allows recording 360° high-resolution (20MP) multi-view stereo videos under diffuse lighting.
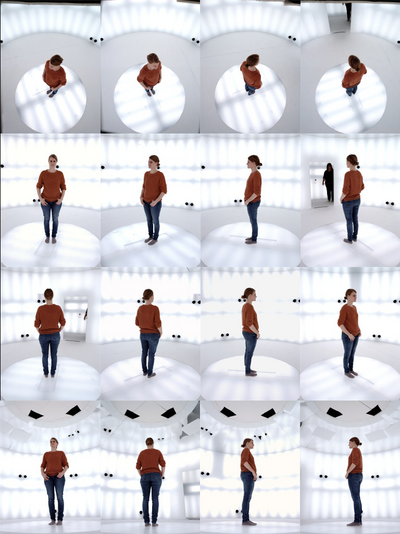
Based on the multi-view data, we reconstruct 360° 3D-geometry of the captured person, which is used to personalize a human template model. After the model adaption and tracking, we reconstruct a static full-body texture from the multi-view footage.
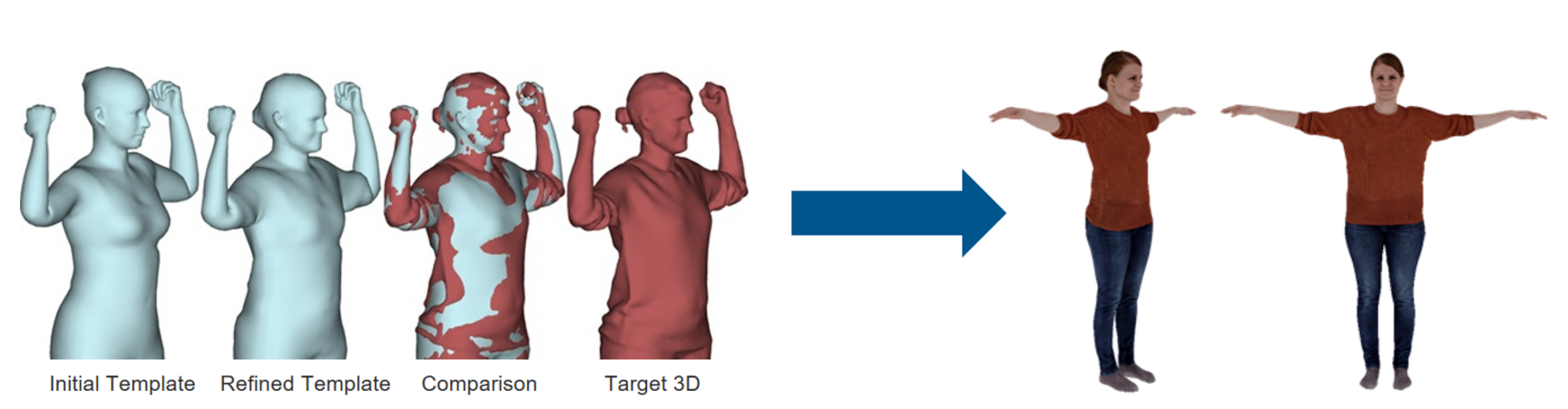
The following video shows an animation example of our avatar:
Facial Animation with Neural Face Models
In order to synthesize realistic facial expressions, we developed a hybrid animation approach. We use an approximate face geometry model that allows capturing rigid motion and large-scale deformations. Fine details and expressions that cannot be represented with geometry alone are captured with dynamic textures. Following this approach, we extract rigid-motion, blend shape-weights and dynamic texture sequences from the captured multi-view video footage.
Based on the extracted face data, we train a variational auto-encoder that is capable of synthesizing face-geometry as well as face-texture from a low dimensional latent code.

Based on this neural face representation, we implemented different animation approaches, which includes regression-based approaches (i.e. prediction of facial animation parameter based on detected landmarks) or example-based approaches (i.e. synthesis of visual speech based on visemes).
The following video shows a viseme-based animation using our neural face model saying “Samstag, Quellwolken und Regen”
Monocular Body Tracking with Temporal and Shape Consistency
In order to simplify the process of human performance capture, we improved an existing approach for monocular human body capture (https://smpl-x.is.tue.mpg.de). By introducing a non-linear refinement step, we are able to enforce consistent body-shape-parameters and apply temporal smoothness to animation parameters. This allows reconstruction high quality animation parameters from un-calibrated monocular video.
The following video shows our avatar being animated from animation parameters that were reconstructed using our monocular approach.
Animation of Digital Humans using Volumetric Data
Apart from classical model-based approaches, we also explored animation-methods based on textured 3D scan sequences. The advantage of 3D scans (volumetric data) is a much higher visual quality, since the appearance of the captured human can be fully reproduced. However, this comes at the cost of missing animation capabilities, since there is no consistent underlying structure. We overcome this problem by performing a key frame-based temporal mesh registration that is able to provide a temporally consistent mesh topology for 3D scan sequences. Using the consistent mesh topology, we are able to perform example-animation of humans by concatenating snippets of captured scan sequences.
The following video shows a sample animation of this concatenative approach, where two scan sequences are connected seamlessly using our temporal mesh registration method.
Project Consortium
- FINCONS
- University of Surrey
- Fraunhofer HHI
- Human Factors Consult (HFC)
- SWISS TXT
- Vlaamse Radio- en Televisieomroep (VRT)
Awards
The project won the NAB 2020 Technology Innovation Award and two of our publications were awarded with the CVMP Best Paper Award 2019 and the CVMP Best Paper Award 2020.
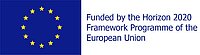